The Role of Data Analytics in Improving Returns Management
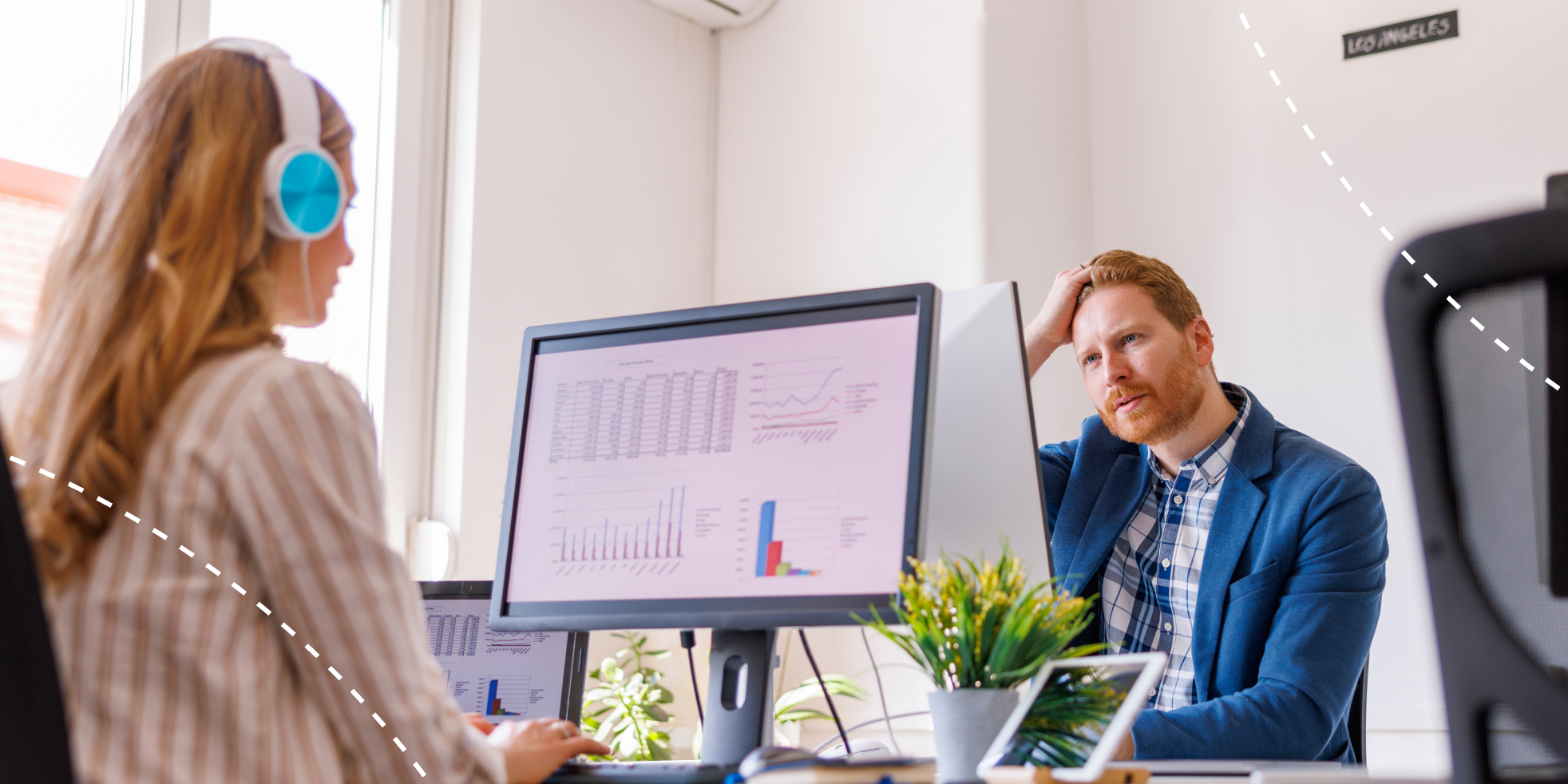
Returns management is a primary pillar of e-commerce operations, tasked with efficiently processing product returns. Given that 15% of online sales will be returned, the process requires major resources and manpower in the e-commerce ecosystem. This means optimizing becomes a priority. One of the main strategies for achieving that is leveraging data analytics.
The growing complexity of e-commerce, combined with rising return rates and diverse customer expectations, has highlighted the need for innovative solutions. Returns management begins when a customer decides to make a return and initiates the process through the online retailer’s portal. Leveraging or implementing data analytics in this process allows the business to use relevant insights to enhance the efficiency of the entire returns process.
Common Challenges in Returns Management Operations
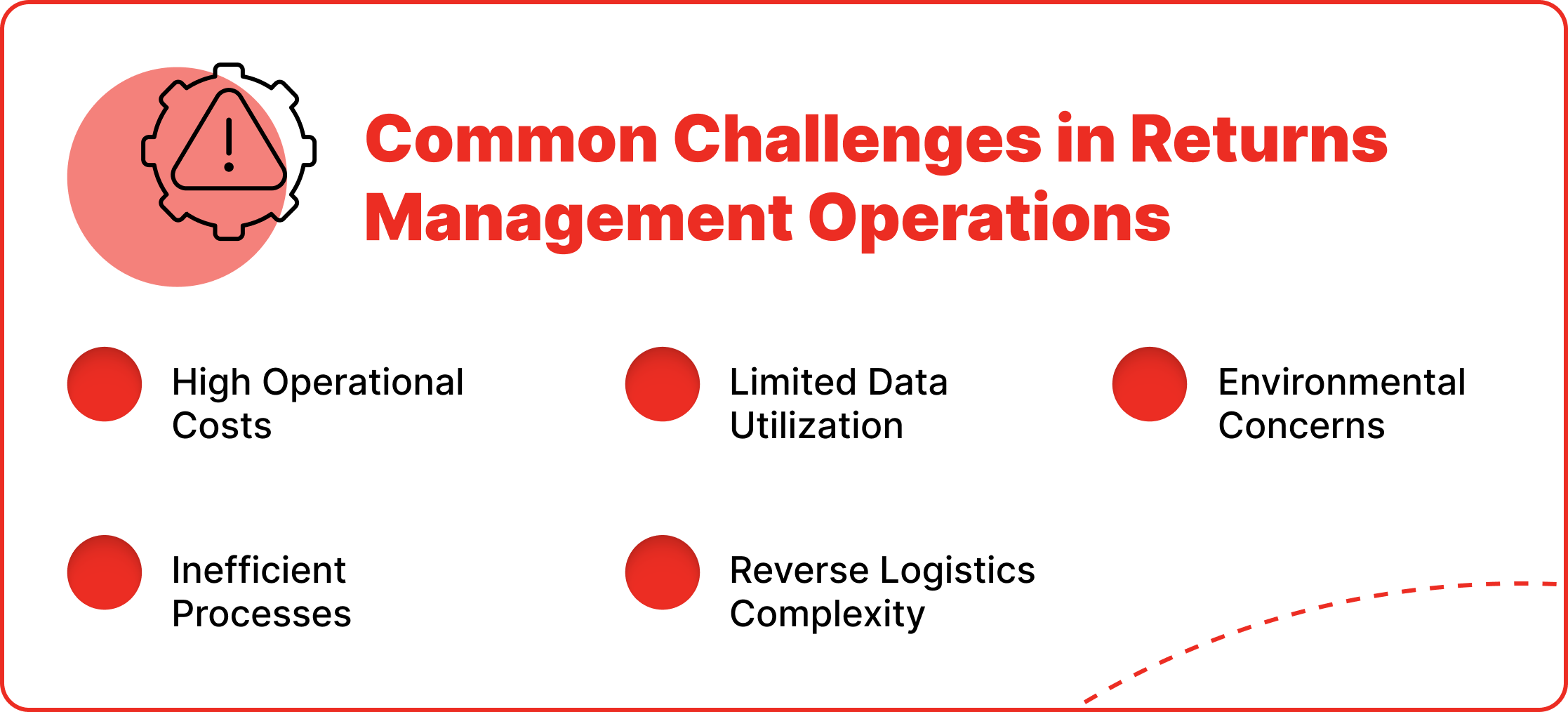
The returns management process is fraught with several challenges impacting profitability, efficiency, and customer satisfaction. Here are some common hurdles companies face:
1. High Operational Costs
Handling returns involves expenses for transportation, processing, restocking, and warehousing. Each step incurs costs that can quickly add up, particularly during peak seasons when return volumes are higher. Additionally, maintaining facilities or staff dedicated to returns can strain budgets.
2. Inefficient Processes
Returns often require multiple steps, including inspection, repackaging, and reintegration into inventory. Without streamlined systems, delays can occur at each stage, slowing the process and creating bottlenecks. This inefficiency not only increases costs but also prolongs the time customers receive refunds or replacements.
3. Limited Data Utilization
Without robust analytics, businesses struggle to identify patterns in returns. This lack of information makes it harder to address root causes, such as product flaws or logistical issues, and limits opportunities for improvement.
4. Reverse Logistics Complexity
Reverse logistics can be incredibly complex, especially when routing returned items to appropriate destinations — whether back to inventory, a recycling facility, or a repair center — requires careful planning. Poor routing increases transportation costs and delays, reducing overall efficiency.
5. Environmental Concerns
Returns can have significant environmental implications, especially when items are discarded instead of recycled or refurbished. Businesses increasingly face pressure to handle returns in a way that minimizes waste and environmental impact, adding another layer of complexity.
The Power of Data Analytics in Returns Management
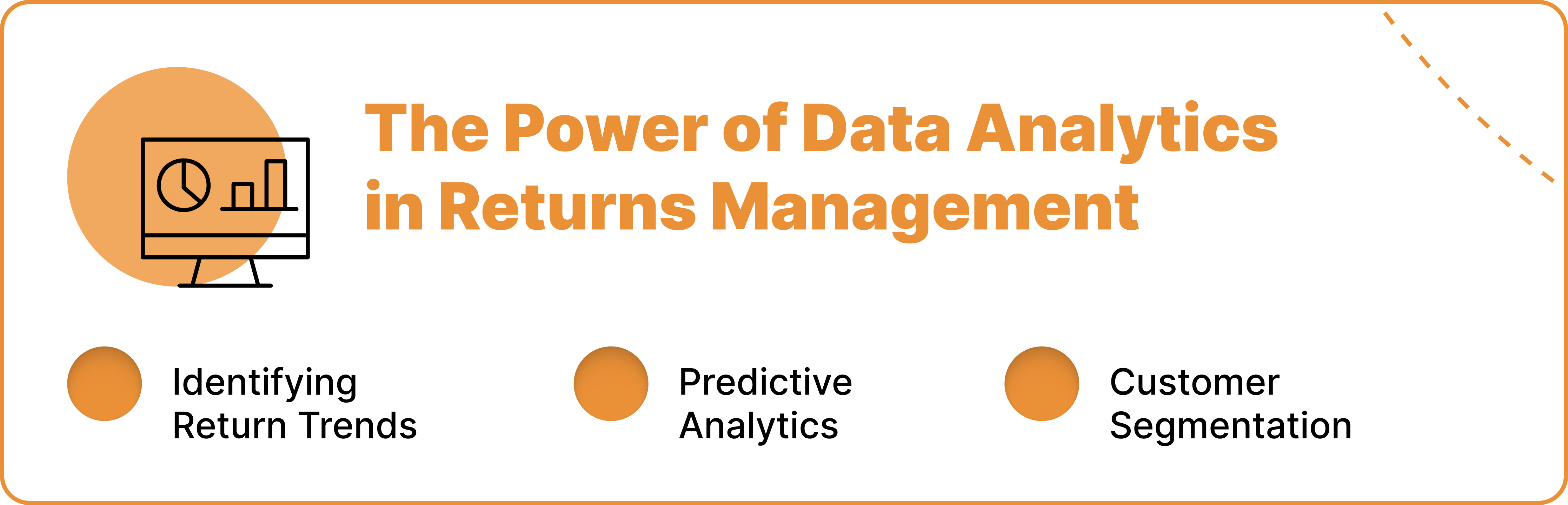
The returns process can be complicated, making optimizing the entire process challenging for the e-commerce business or online retailer. However, with data analysis, that becomes more streamlined. Here is how:
1. Identifying Return Trends
Analyzing return data helps businesses uncover patterns that would otherwise go unnoticed. Common return reasons, such as sizing issues or defects, can inform product improvements. Identifying peak return periods enables better resource allocation during those times, ensuring that staff and facilities are prepared for higher volumes.
2. Predictive Analytics
Forecasting future return volumes using predictive analytics allows businesses to anticipate challenges before they arise. By studying historical data, companies can prepare for seasonal spikes or anomalies that might disrupt operations. For instance, retailers can adjust inventory levels or allocate additional staff to handle expected returns efficiently.
3. Customer Segmentation
Segmenting customer’s data based on their return behavior can lead to more targeted policies. High-value customers might benefit from enhanced return experiences like free shipping or extended return windows. At the same time, frequent returners could be guided toward better purchasing decisions through detailed product descriptions or sizing guides.
Key Metrics To Track in The Returns Management Process
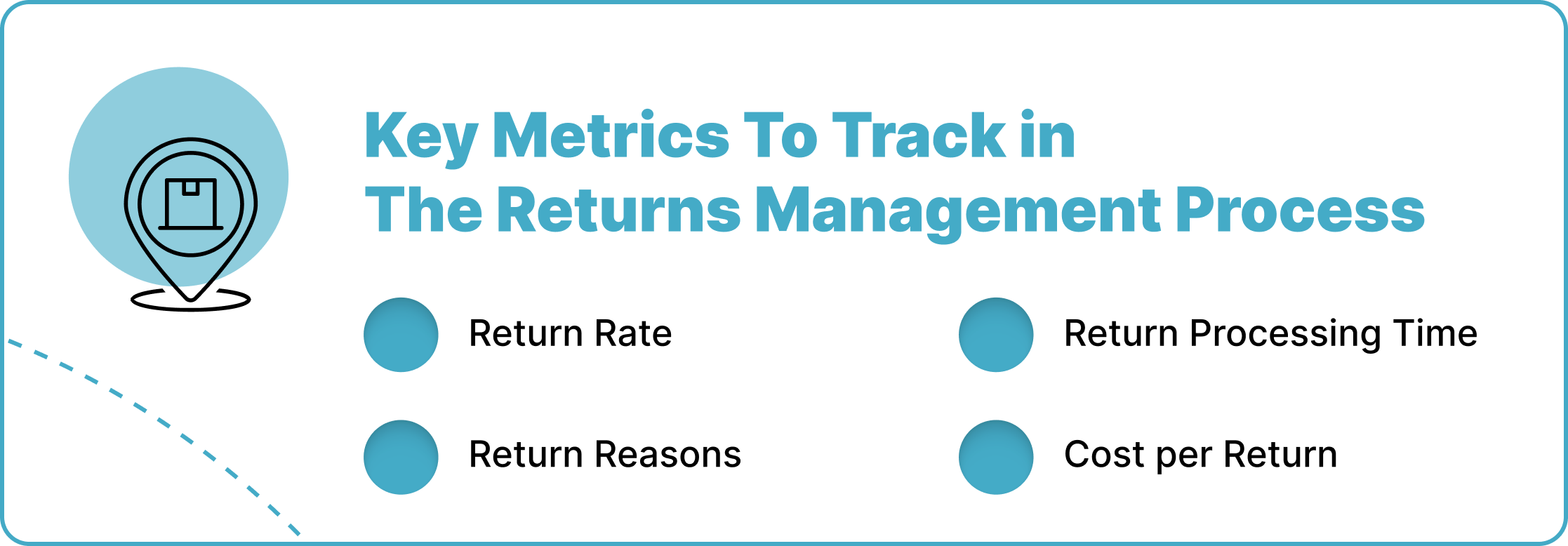
When leveraging data analytics in the returns management process, certain metrics or returns data must be closely monitored for relevant insights. This allows for more process optimization.
1. Return Rate
This metric indicates the percentage of items returned out of total sales. Monitoring trends in return rates can reveal whether recent initiatives — like improved product descriptions or packaging changes — are effective.
2. Return Reasons
Understanding why customers return products helps pinpoint areas needing improvement. Identifying and addressing these reasons can reduce future returns, whether it’s a sizing issue, inaccurate descriptions, or damaged goods.
3. Return Processing Time
The speed at which returns are processed is directly linked to customer satisfaction. Shortening processing times requires efficient workflows and technology, ensuring faster refunds or replacements.
4. Cost per Return
Calculating the cost associated with each return — covering transportation, restocking, and labor — highlights areas where efficiency can be improved to save money.
How to Implement Data-Driven Strategies
Although the role of data analytics in returns management operations cannot be understated, it is important to implement it properly for the best results. To do this, consider the following strategies:
1. Leverage Analytics Tools
It is important to leverage the right platforms or tools to get the most out of data analytics in the returns management process. These data analytics platforms can help the e-commerce platform process vast amounts of return data and provide actionable insights that can be used to streamline and optimize the entire process. These tools also enable the online retailer or e-commerce business to leverage data visualization, track metrics, uncover patterns, and identify inefficiencies in real time.
2. Collaborate With Cross-Functional Teams
Returns data shouldn’t exist in a vacuum. It should be shared with other reverse logistics chain stakeholders, including departments and suppliers. Share insights with customer service, product development, and operations teams to ensure a coordinated approach to addressing root causes and implementing solutions. For instance, product development teams can use return feedback to improve designs, while customer service teams can refine their communication strategies.
3. Continuous Improvement
For best results, it is important for the returns management team to regularly review the return metrics to see if they are still relevant to the business. Refining processes when needed is vital to ensure that strategies remain effective. Implementing data-based iterative improvements can lead to sustained reductions in return rates and enhanced customer satisfaction.
Simplify Data Analytics in The Returns Management Process With ReverseLogix
ReverseLogix is built with meaningful metrics and game-changing insights in every module. With end-to-end returns management and best-in-breed tracking and data analytics techniques. The platform is the solution to unite all your existing business technologies and deliver metrics and data to every team member. Gain total visibility across the entire returns journey, optimize performance for enhanced competitive advantage, and increase your edge in real-time. Enjoy all amazing benefits such as diagnostic analytics, descriptive analytics, customer feedback, data storage, and detailed customer feedback through a real-time platform that can collect and analyze raw data. Put ReverseLogix in your hands and unlock more value from every return. Connect with us for a free demo here.
Frequently Asked Questions
Data analytics helps businesses understand patterns in return behavior, predict future return volumes, and identify common reasons for returns. This information enables organizations to create strategies that reduce the frequency of returns and improve operational efficiency.
By collecting data management and examining them on product categories, customer demographics, and timing, businesses can spot recurring issues, such as defects in specific products or seasonal surges in returns.
Predictive analytics, combined with statistical analysis of the entire returns process, can forecast return rates, allowing businesses to prepare inventory and staffing levels accordingly. This reduces delays, enhances resource allocation, and minimizes costs.
1